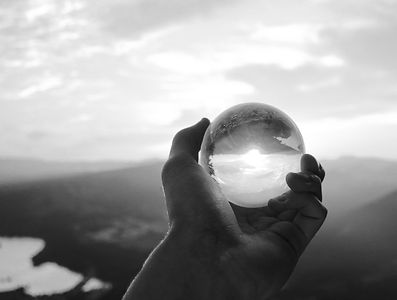
Heading 6
Predictive analytics
What is predictive analytics?
​
Predictive analytics is the practice of aggregating and analyzing historical data to anticipate future outcomes. Aggregating multiple datasets connects the dots between different departments, business processes, and types of data (structured vs. unstructured).
​
However, simply aggregating various data points does not necessarily indicate future behavior. Predictive analytics leverages statistical techniques like data modeling, machine learning, and even artificial intelligence to uncover patterns in big data.
While these patterns cannot predict exactly what will happen in the future, predictive analytics can identify trends, herald disruptive industry changes, and allow for more data-driven decision making.
​
Practical applications of predictive analytics
​
Any field that captures data is a candidate for predictive analysis. Everything from enhancing cybersecurity to developing more targeted marketing to strengthening actuarial performance is fair game.
​
Predictive analytics in healthcare
​
Healthcare is a primary use case for predictive analytics. A major issue in healthcare is the difficulty of predicting patient risk. Actuarial teams need to establish optimal insurance rates and governmental requests for reimbursement for members with various health issues.
​
Due to this need, health insurance agencies were some of the first companies to adopt big data practices. Actuaries use predictive analytics to determine things like a patient’s predisposition for developing a worsened condition, or a patient’s likelihood of participating in sponsored wellness activities.
Predictive analytics allows health insurance companies to examine patterns of risk among patients of similar age, with similar conditions, and from similar social determinants of health. Armed with this information, health insurance companies are able to make more informed financial and ethical decisions.
Predictive analytics in finance
​
Lending, a key function of the financial services industry, has been revolutionized by predictive analytics. Before a bank gives out a loan, they want to make sure that a customer is trustworthy. Ultimately, they want their money back. So how do underwriters gauge that trust?
​
Until several years ago, underwriters would judge an applicant based on past performance and personal hunches. Underwriters would review the applicant’s history and debt-to-income to arrive at a convoluted interest rate. As new financial laws emerged, lenders had to develop a more statistically relevant method for underwriting.
The lending industry underwent a revolution when third-party predictive analytics models like VantageScore and FICO Score became available. These models allowed lenders to calculate accurate risk-based interest pricing, and limited subjective bias. Instead of basing interest rates on a few outdated metrics, the VantageScore and FICO Score models are based on the performance of millions of borrowers with similar spending tendencies.
​
How predictive analytics works
​
Predictive analytics seems like magic, but it stems from statistical science. At its core, predictive modeling involves giving the presence of particular variables in a large dataset a certain weight or score. This score is then used to calculate the probability of a certain event occurring in the future.
​
There are two main statistical modeling approaches used in predictive analytics: classification models and regression models.
Classification models
​
Classification models are typically binary. For example, one company might be interested in member enrollment. A classification model will tell you if a member is likely to either stay with the company or disenroll in a given timeframe, based on certain criteria.
​
Regression models
​
Regression models are less black and white. Instead of a 0 or 1, regression models will predict an actual number. Consider an example in healthcare: let’s say a member has a BMI of 29. A regression model might predict that the member’s BMI could drop 3 points in the next year with a consistent, healthy diet.
​
​
Techniques for predictive analytics
There are several techniques data scientists use to construct classification and regression models. Namely, decision trees, regression, and neural networks.
-
Decision trees visually represent a path of choices. Each branch of the decision tree is a possible decision between two or more options, whereas each leaf is a classification (a yes or no). Decision trees are one of the more attractive techniques for modeling because they can handle missing values and are simple to comprehend.
-
Regression is another popular modeling tool. As discussed earlier, regression is used with continuous data as opposed to binary data. Different data questions require different applications of regression. For instance, linear regression is used if only one independent variable can be ascribed to an outcome. If multiple independent variables have an effect on an outcome, multiple regression is most appropriate. Logistic regression is an even more complex form of regression that does not follow the same convention as linear and multiple regression. Unlike the other two regression models, logistic regression is used when the dependent variable is binary. For example, a logistic regression could be used to evaluate how the odds of a patient having a heart attack (binary variable) change with every additional BMI value (continuous variable).
-
Neural networks represent the final, most complicated technique. This method is becoming more and more in-demand because perfectly linear relationships are rare in nature. Neural networks allow for more sophisticated pattern recognition by employing artificial intelligence.
Although these statistical methods are not new, they are being more widely accepted and used. This can be attributed to the rise in popularity of the cloud.
​
Big data, the cloud, and the future of predictive analytics
​
Before the cloud, predictive analytics seemed impossible. Computers did not have the capacity to house petabytes of data, let alone have enough processing power to run labyrinthian data models. The cloud offers companies a way to compile and combine multiple, huge data sets and easily scale their models.
​
There are many emerging, cloud-based predictive analytics products. In the future, the cloud will enable companies to build their own machine learning models. By teaching a computer to find patterns in the data, the cloud eliminates manual work and allows for greater interpretation and extrapolation.
​
The cloud also allows for more customization and flexibility. With the advent of internet of things (IoT)on the cloud, predictive analytics tools could get even more granular in their assessment of people’s everyday habits.
​
Modern predictive analytics software and tools
​
Now that companies are able to readily retrieve large datasets from the cloud, there is so much room for big data analysis, and there are many cloud-based predictive analytics software options on the market. While it is vital to have a team of experts to interpret data models, software is necessary to lessen the time to collect, clean, and analyze data. Predictive analytics software can digest both stored and real-time data, and assist in appropriate formatting.
​
In addition, most cloud-based predictive analytics software integrates well with ERP systems, digital analytics software, and business intelligence platforms that most companies already have. Business intelligence teams can also use predictive analytics software to demonstrate the value of predictive analytics in visual form via dashboards.
See what independent research firms are saying
Gartner® recognizes Microsoft as a Leader for the fourteenth consecutive year in the 2021 Gartner Magic Quadrant™ for Analytics and Business Intelligence Platforms.1
Forrester recognizes Microsoft as a Leader in The Forrester Wave™: Augmented BI Platforms, Q3 2021.